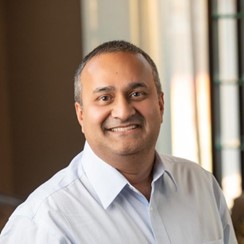

The healthcare trade is experiencing an information and technological revolution that’s accelerating drug discovery and supply to sufferers. Over the previous 15 years, we’ve seen an explosion of focused therapies — together with small molecules, immunotherapies, cell-based therapies, and extra — all made attainable by the combination of large-scale ‘omics knowledge with medical insights, remedy approaches, and affected person outcomes. Nevertheless, regardless of these developments, we nonetheless have an extended technique to go to completely understand insights gained from these breakthroughs with a view to drive well timed discoveries. Underscoring that is that there are over 7,000 uncommon illnesses, but solely 500 have authorized therapies. In oncology, a big lengthy tail of much less widespread most cancers sorts continues to current distinctive challenges for analysis, analysis, and remedy. Furthermore, the advanced genetics underlying widespread illnesses stay largely unexplored, with a lot work wanted to completely translate these insights into holistic enhancements in affected person care.
In an period the place new knowledge streams are continually rising, there may be an pressing want for streamlined platforms that may seamlessly combine the most recent population-scale and proprietary datasets, together with medical, information, and multi-omics knowledge. This entry to different knowledge sources, along with a various set of instruments and workflows, will enable researchers to research advanced knowledge in actual time, determine potential drug targets, and ensure potential therapies quicker. This can be achieved by means of novel knowledgeable methods, metadata and knowledge pushed mapping to applicable analytics, and low-code options that help in streamlining sophisticated processes, decreasing guide steps, and accelerating analysis timelines. General, these developments put drug growth into double time, with out risking high quality as a result of ongoing rigorous validation, thus catalyzing adoption of novel therapies as the usual of care.
The Promise of AI and Massive Language Fashions
The healthcare trade has seen a increase in using synthetic intelligence (AI) and huge language fashions (LLMs), that are already altering how precision drugs is utilized. AI is instrumental in recognizing patterns inside advanced datasets, enhancing interrogation accuracy, and facilitating the exploration of enormous volumes of medical and ‘omics-based analysis knowledge. LLMs, with their potential to research and generate human-like textual content, provide important potential for compiling medical information, decoding analysis papers, and making predictions primarily based on medical knowledge, permitting the analysis skilled to work together with the system conversationally to start out the method.
But, the complete potential of those instruments can solely be realized when AI and LLMs are built-in right into a unified and standardized analytics and insights platform that analyzes uncooked knowledge/alerts to determine discrete analytes equivalent to variants, expression profiles, and protein ranges after which derive insights from these analytes utilizing superior AI algorithms and annotations together with these that could be derived utilizing LLMs.
When related, these applied sciences can analyze massive datasets, infer illness mechanisms and pathophysiology, and make clear traits, providing insights into potential future outcomes that may have in any other case been missed, finally driving analysis and accelerating the trail to new therapies. Higher leveraging of data- and conventional analytics-driven analysis ecosystems with AI and LLMs can pace up the combination of promising therapies into commonplace care.
Overcoming Challenges in AI and LLMs Integration
Regardless of the potential of AI and LLMs, their integration into healthcare isn’t with out challenges. Researchers and healthcare suppliers want to concentrate on the next:
- Information High quality and Integration: Use of curated knowledge, equivalent to annotation databases and analyzed multimodal datasets, with unstructured knowledge, like medical notes or analysis papers, as the idea for making inferences by LLMs is vital to the final word accuracy of the outcomes returned. Your entire analytical toolkit, together with AI algorithms, LLMs, clustering/classification instruments, biostatistical strategies, and superior computational strategies, must be used collectively intelligently for particular person analysis or medical contexts to make sure enough specificity and sensitivity.
- Digital Hallucinations: Hallucinations happen when LLMs produce inaccurate info, a critical difficulty in healthcare. “Match-for-purpose” fashions, that are fine-tuned with curated knowledge or guided by prior information, enhance accuracy and cut back the chance of such errors by specializing in particular duties.
- Mannequin Transparency: Tailor-made fashions, which give the underlying proof resulting in observations returned, enable customers to hint outcomes again to references which can then be verified by area consultants. That is vital for making fact-checking extra simple in comparison with generalized fashions.
Information Safety and Privateness
With the expansion of AI and LLMs in medical settings, knowledge safety and affected person privateness are extra vital than ever. In 2024 alone, over 300 cyberattacks on healthcare methods had been reported, highlighting the vulnerabilities inherent in dealing with delicate medical knowledge. To mitigate these dangers, healthcare organizations have to implement strong knowledge safety protocols, together with:
- Anonymizing Affected person Data: That is the method the place private info is faraway from medical knowledge earlier than its use in AI methods such that the information will not be used to reidentify the person. This ensures affected person privateness whereas permitting AI to research knowledge, determine patterns and produce predictions, supporting analysis with out compromising affected person privateness or security.
- Establishing Devoted Networks: Like controlling their digital environments on premise, researchers and precision drugs corporations ought to set up their very own devoted cloud networks. By leveraging a personal and safe cloud infrastructure, delicate knowledge may be processed, saved, and accessed with a better sense of management.
- Establishing personal environments for AI/LLMs : Precision drugs corporations and analysis establishments ought to be certain that proprietary fashions primarily based on commercially obtainable LLMs don’t enable for unauthorized use of knowledge by the LLM supplier and {that a} model managed, non-modifiable native copy of the LLM is used previous to utilizing strategies equivalent to immediate engineering or positive tuning to create application-specific fashions. These distinctive fashions ought to function in remoted environments to forestall knowledge publicity, with strict model management and validation to make sure their accuracy and reliability over time. Common validation helps preserve consistency and prevents errors or integrity points.
Amidst these technological greatest practices, there does stay another element that isn’t know-how primarily based. That lacking piece is the human contact, which stays an important factor to the institution of a platform constructed on harnessing AI and LLMs.
The Human Contact
Regardless of the more and more unbelievable capabilities of AI and LLMs, they can not exchange the crucial position of healthcare professionals and researchers. The “human contact” stays important for decoding AI findings, making use of insights within the context of particular person sufferers, and guaranteeing that requirements are upheld.
By creating collaboration between human expertise and experience, with know-how’s skills to eat intensive portions of knowledge, a synergy may be shaped that accelerates the interpretation of discoveries into medical observe whereas guaranteeing that prime high quality affected person care stays on the middle of the method.
Transferring in Double Time
The normal tempo of medical discovery is way too gradual for at present’s fast-moving healthcare panorama. By harnessing a complete know-how toolkit together with AI and LLMs and incorporating the human contact, all inside a safe data-sharing platform, these throughout the precision drugs trade can see firsthand accelerated scientific breakthroughs attain sufferers and start to create constructive impacts sooner.
About Rakesh Nagarajan, MD, Chief Medical Officer at Velsera:
Dr. Rakesh Nagarajan is the Chief Medical Officer at Velsera, specializing in democratizing medical genomics and advancing precision analysis by means of ‘omics applied sciences. With almost thirty years of expertise on the intersection of pc science, informatics, and drugs, he’s a skilled doctor scientist dedicated to medical and translational analysis.